When will quality assurance with AI be available?
Mr. Spring, everyone is talking about AI today. But what is AI and how does it differ from machine learning?
We speak of AI when the tool behind it is capable of making autonomous decisions that match or exceed human intelligence. In doing so, the AI tool is designed to constantly learn and adapt in order to make better decisions tomorrow than it does today.
For AI to achieve this goal, it needs large amounts of data. This is complemented by the use of advanced algorithms and models to analyse large amounts of data, identify patterns and derive meaningful insights. Unlike traditional data processing, AI systems can handle complex tasks, solve problems and have an intelligence that enables them to respond to different scenarios in a situation-specific manner. AI therefore stands for intelligent decision-making and problem-solving capabilities that go beyond mere data processing. AI has the ability to reason and adapt to new situations - which in turn also allows it to find novel solutions that have not been predetermined.
... and what characterises machine learning in contrast to this?
In machine learning, we're talking about a part of AI that focuses on the development of algorithms and statistical models. Machine learning enables computers to learn from existing data and thereby improve their results. This usually focuses on one specific application. A very illustrative example here is the topic of speech recognition systems, which we encounter almost daily today: This kind of system may sound intelligent to the user, and to the layperson it may seem obvious that it is artificial intelligence. However: The speech recognition system has no understanding of the content of the speech. It only listens for key sounds and then carries out the predefined tasks. The machine learning algorithms used here are designed to learn iteratively from the available data in order to continuously improve the results obtained from the algorithms.
Can you give us examples of machine learning in the food industry?
This is, for example, the function for predictive maintenance of production machines. Within these systems, machine data is analysed in order to predict potential failures and to optimise the timing of the replacement of, for example, wearing parts in such a way that the necessary downtime can be reduced.
As a provider of product inspection solutions, we at Mettler-Toledo have been using this kind of machine learning for many years, for example in our X-ray inspection systems, in order to minimise the number of false rejections. The inspection system is trained with good products, so to speak, in order to optimise the detection accuracy between defect-free and defective products.
In summary, this means: Machine learning focuses on training models on data to make predictions or perform tasks. Machine learning is one aspect of AI, but it encompasses much more than just learning from data.
What are the advantages of both technologies for the food industry?
Both AI and machine learning offer many opportunities. Machine learning enables predictive maintenance that optimises machine performance and reduces costly downtime. The use of AI opens up new opportunities to improve food safety both in production and in logistical processes along the supply chain. This sustainably increases the productivity of the food manufacturer. Digital food safety solutions in particular hold immense potential for the integration of artificial intelligence, for example for digital track & trace transparency "from farm to fork". Furthermore, the seamless integration of AI into existing systems and applications enables comprehensive data analysis and more informed decision-making - both with regard to possible efficiency increases and the optimisation of automation processes. Companies can also use artificial intelligence to automate tasks that were previously carried out manually. At the same time, it is important to train employees on how the integration of AI systems can support them in their everyday work and how it can be integrated as efficiently as possible.
Although AI shows great potential, it also comes with risks and challenges. The data quality of the data fed into the AI systems is crucial for all subsequent processes. The GIGO principle applies here: Garbage in? Garbage out! Incorrect data quickly leads to incorrect decisions as a result. Any small error or malfunction in one part of the system can have cascading effects when AI systems operate fully autonomously. That's why human monitoring and the implementation of safeguards are essential for reducing such risks.
How far away are we from the introduction of AI in the food industry?
AI is already experiencing its first implementations in high-end systems and applications. However, it currently has no significant impact on production lines in practice. However, as technology advances and connectivity improves, the potential for AI to transform operational processes is sure to increase. By integrating product inspection into AI systems, comprehensive data from multiple applications, devices and processes can be accessed in perspective, leading to more informed decisions. Our product inspection technology provides a wide range of data on food production processes such as quality control, foreign object detection and packaging integrity. This amount of data can be analysed by AI algorithms to identify patterns, predict outcomes and improve various aspects of food production. For example, AI can use this data provided by our product inspection systems to optimise energy consumption, identify environmental impacts and create predictive maintenance plans to improve overall production efficiency.
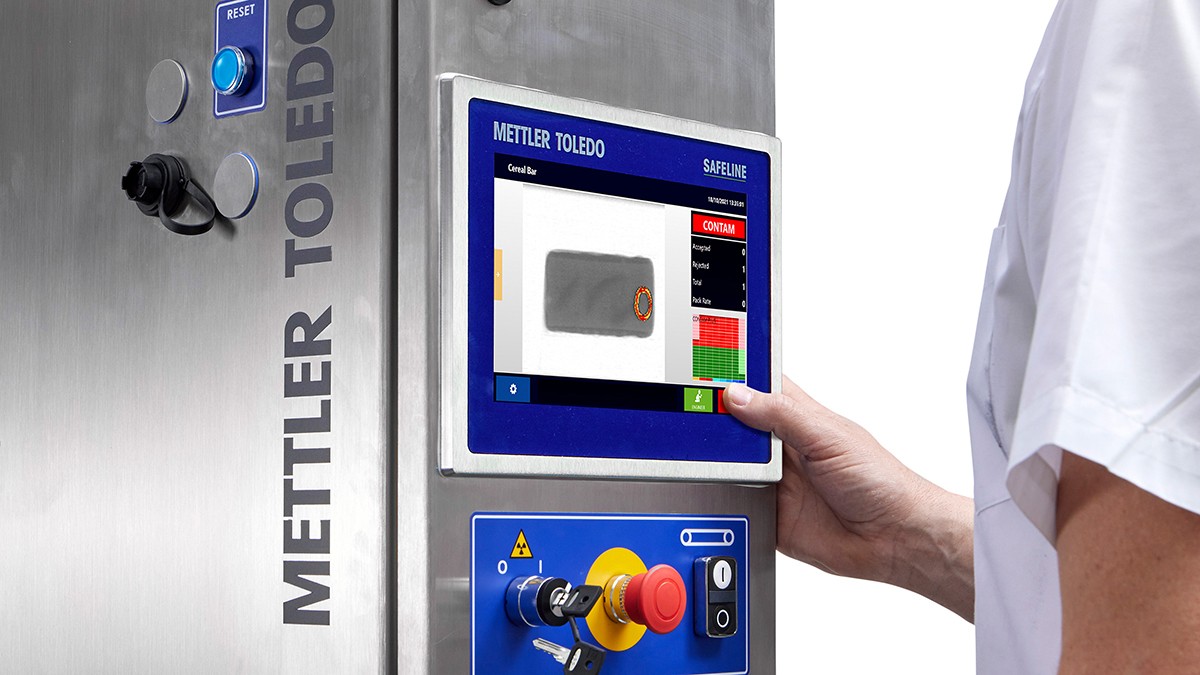
Product inspection solutions, such as X-ray inspection systems, use machine learning to minimise the number of false rejections during foreign object detection. @Mettler-Toledo