Digital Twins and Machine Learning
What is the aim of your project and why is it relevant for the food industry?
Christian Krupitzer: The aim of our project is to investigate the potential of digitalisation in the food industry, particularly for dairies. In doing so, we're guided by digitalisation in other industries (e.g. the automotive industry) and apply these findings to food production in order to demonstrate its digitalisation potential. We analyse both the data that has already been collected and the ways in which it can be used. And we investigate what additional data is needed to realise the vision of Industry 4.0 in the food industry as well. A particular focus is on anomaly detection. We consider production data from both fermented and non-fermented dairy products to account for variances and different production steps and machine types. Our aim is to use machine learning to identify anomalies in the data and to check whether these anomalies can predict fluctuations in product quality or potential processing problems. Consequently, we want to use digital twins and machine learning to make production processes in the food industry safer and more efficient.
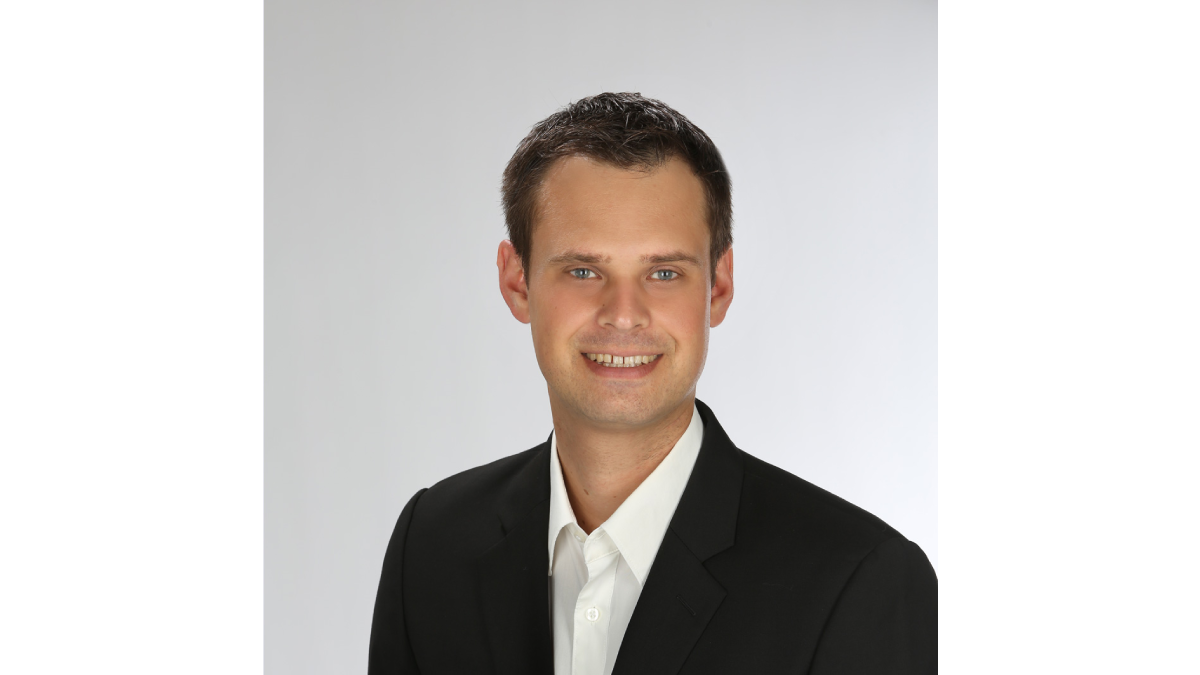
Jun.-Prof. Dr. Christian Krupitzer, Head of the Department of Food Informatics ©Universität Hohenheim
What data is collected for the project and how do you support quality assurance?
Dana Jox: The collection of a wide range of data is essential for our project. We work with machine data as well as food data and higher-level production information. This data helps us to predict anomalies and to better understand the condition of the products. The data collected includes:
- Machine data: This is used in particular to predict anomalies. Here we record parameters such as temperature, pressure and machine running times.
- Food data: This describes the condition of the product and includes quality parameters such as moisture, fat content and pH values.
- Production data: This includes information on the production process, for example product changes or the influence of employees on the production line.
Our data collection focuses not only on the end product, but also on the processes and influencing factors along the entire production chain. As a result, we can predict specific anomalies that could affect product quality.
This comprehensive data collection is crucial for quality assurance. It enables us to identify potential problems at an early stage, especially those that arise from complex and parallel production processes. The data provides us with valuable insights into the various factors influencing the production process or product.
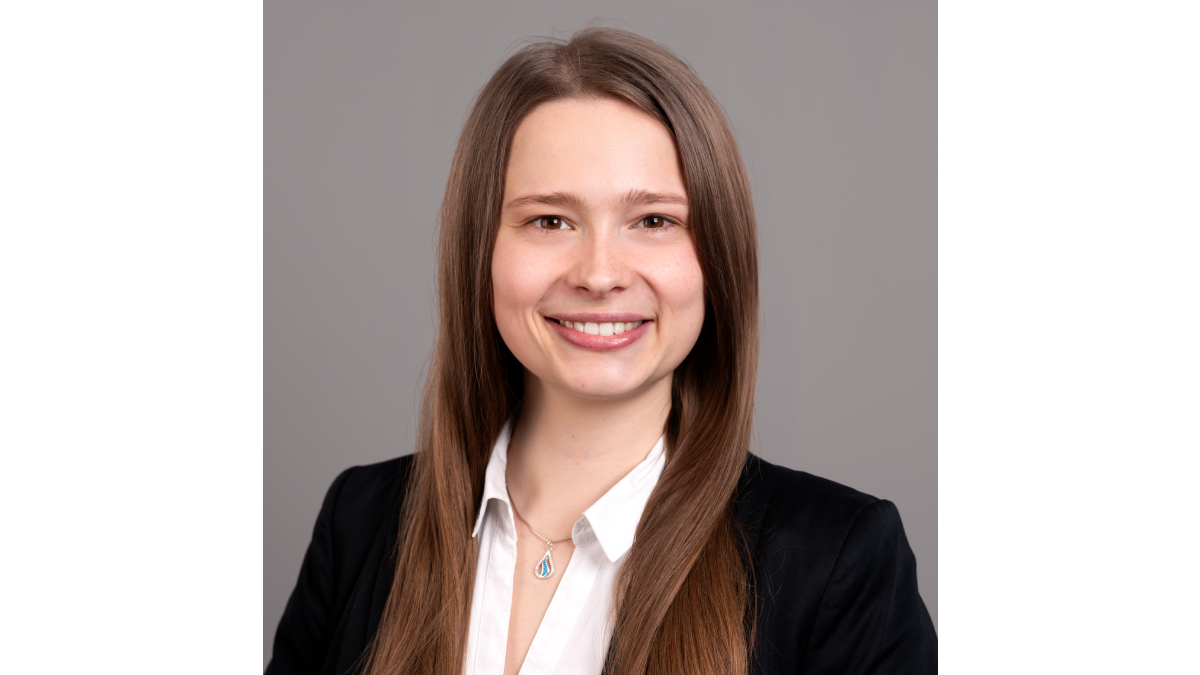
Dana Jox, M.Sc., PhD student and research assistant in the project ©Universität Hohenheim
What specific advantages do digital twins offer in food production?
Christian Krupitzer: The digital twin is a virtual representation of a real object or process that allows us to simulate and analyse different scenarios without having to intervene in actual production. In food production, it offers numerous specific advantages, although its application in this industry is more challenging than in the automotive industry, for example, due to the complexity of biological processes.
Digital twins are already well established in some industries, for example in the automotive industry. In this industry, the entire production process is digitally mapped. By simulating and controlling machine behaviour, predictions can be made about the end product and production can be optimised. This provides accurate, continuous monitoring.
This is complicated in the food industry due to the complexity of the product. In particular, complete monitoring is more difficult in biological processes such as fermentation. For example, it is not possible to measure a 50,000-litre fermentation tank in all respects at all times. This is where the digital twin comes into play, integrating scientific models and assumptions to fill these gaps and gain a better understanding of the processes.
The extended possibilities for using the digital twin in food production include:
- Process modelling: Measurable parameters such as temperature or stirring speed can be modelled in the digital twin. This helps us to gain better insights into processes such as fermentation by adding scientific models based on the measured data.
- Process optimisation through simulation: With a digital twin, we can simulate and optimise production parameters without interrupting the actual production process. This enables us to make adjustments quickly and efficiently, while ensuring the best possible product quality.
In the long term, the digital twin offers considerable potential:
- Adaptation to raw material variability:Simulations using digital twins can help to compensate for fluctuations in raw materials. One example of this is summer and winter milk, which has a different raw material composition. The digital twin can predict these differences and adjust the production process accordingly.
- Product development: The digital twin could also play a key role in product development. For example, it could help predict the effects of recipe changes on flavour and aroma before expensive and time-consuming physical testing is carried out.
- Technology transfer: Another interesting possibility is to transfer findings from smaller pilot plant trials to larger production lines. This speeds up the introduction of new products and processes.
Of course, we're still at the beginning of this technology, and there are still open research questions. Nevertheless, we're confident that the importance of the digital twin in food production will increase.
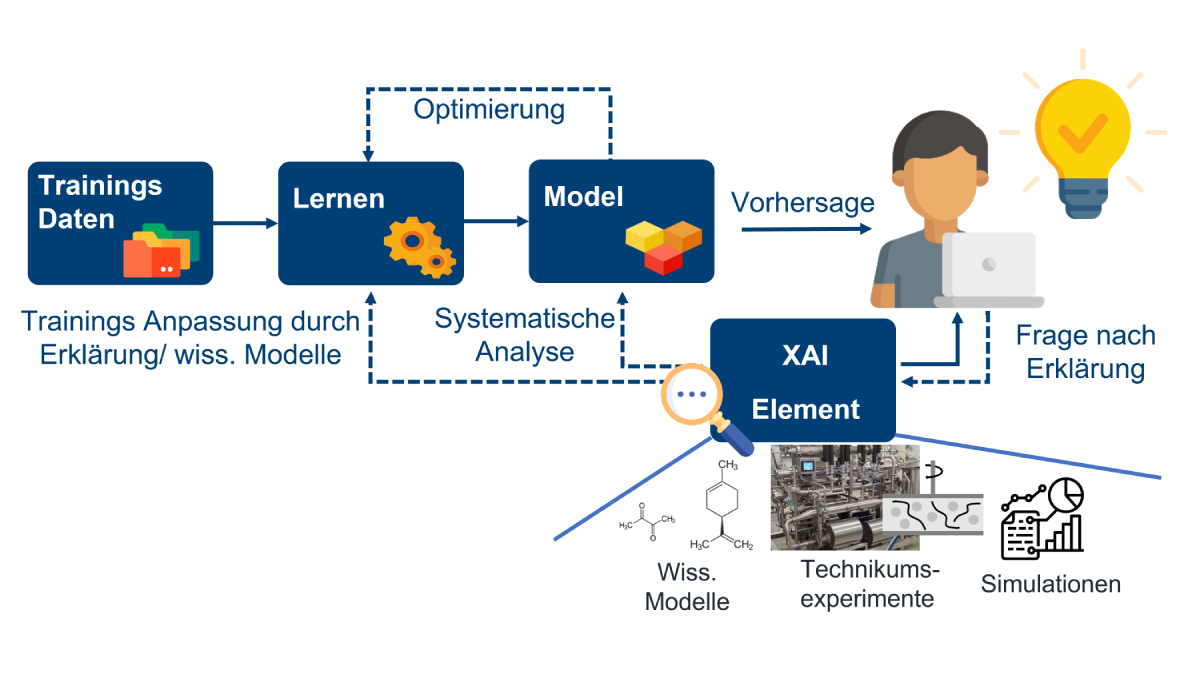
Process mapping through machine learning. ©Jox, Dana., Hummel, D., Hinrichs, J., and Krupitzer Christian.
What are the biggest challenges in introducing digital twins and machine learning into food production?
Christian Krupitzer: One of the biggest challenges is that every manufacturing company has unique requirements and circumstances. Particularly in the food industry, for example in milk processing, production environments vary greatly. There are no universal solutions that can be applied to all companies. This means that each machine learning model has to be customised for a specific production line or product. As the resulting model is very specific to the respective application, we'll develop a machine learning pipeline as part of our project that can be applied to the basic steps of learning a specific model.
Another major challenge is data quality. The creation and maintenance of a machine learning model requires an enormous amount of data preparation. It must be ensured that the data is complete, correct and of high quality. We often have to deal with incomplete or inaccurate data that needs to be carefully processed before it can be used for the model.
Dana Jox: In practice, this process requires the development of an extensive data processing pipeline. This pipeline involves several steps, from analysing the data to handling missing or corrupted records and selecting the appropriate algorithms for machine learning. While some of these steps can be automated and adapted for other production contexts, the data itself often remains specific to the respective company and product. First of all, a comprehensive collection of data in the process and the possibility to retrieve it is fundamental. These two requirements are still not met in all companies.
In addition, many companies in the food industry – especially smaller or more traditional companies – have not yet implemented Industry 4.0 solutions. Resistance to new technologies is often encountered here, making the introduction of AI-supported processes more difficult. In particular, only the implementation costs are initially considered, as the potential of data analysis is still largely unknown. Openness and willingness are needed to implement these new processes, and at the same time, customised solutions must be developed for each company.
What long-term opportunities do you see in the use of digital twins and machine learning in food production?
Dana Jox: In the long term, the use of digital twins offers enormous potential for food production. A key advantage lies in a deeper understanding of production processes and product qualities. The digital twin can help to identify why a product may not have the desired properties or why a process is not running optimally. This in-depth process understanding helps to improve product quality and to make process control more precise.
Christian Krupitzer: Another long-term goal is traceability along the entire production chain. With a digital twin, it might be possible to monitor each individual production stage, enabling conclusions to be drawn all the way up to the consumer. The idea of an adaptive best-before date that is based on the specific production conditions is particularly exciting. The digital twin could perform a dynamic calculation based on the data collected, adapting to factors such as storage, transport and production conditions.
The digital twin also offers great potential in the area of recipe development, particularly in the production of plant-based alternatives. A common problem with plant-based proteins, such as pea proteins, is their taste. The digital twin could use simulations to show how to treat or mask this taste in a targeted manner. This could accelerate the development of new, tasty plant-based products.
In terms of the technological basis, we use AI and machine learning to enable data processing and analysis. Machine learning allows us to recognise patterns in the collected data and make predictions on this basis. This gives us the opportunity to run through various scenarios to assess the impact on the end product.
Generally speaking, we see the digital twin as a key technology that could play a central role in food production in the future. The combination of process optimisation, increased traceability and better product development makes it an extremely promising tool for Industry 4.0.